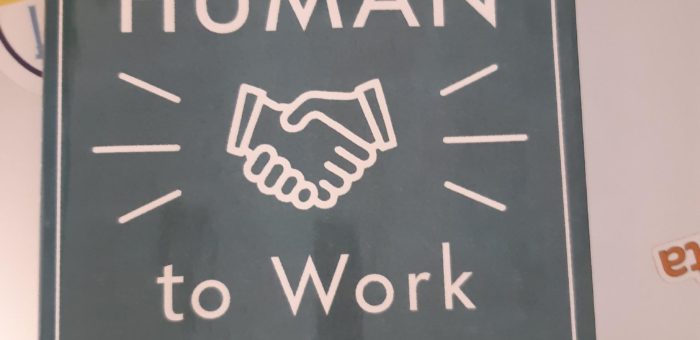
Key learnings from the book “Bring your Human to work” by Erica Keswin
When I was looking for the first book to read as part of my one book per month goal in 2020, I came across "Bring your Human to work" and it caught my attention, read its summary and reviews, and I decided to buy it and I can say after finishing it, that I made a great choice. I loved going through its chapters, it's easy to read and it contains a "Human action plan" at the end of each chapter. It's about bringing the best of ourselves to work, and the best of our employees if we are entrepreneurs. At work, many times the human part is neglected, because often the focus is more on the business, but the research shows that when companies focus on their employees first…